- Published: 2025-04-10
- Updated: 2025-05-26
- MeshLib Team
3D Mesh Decimation Library Comparison
Key Takeaways
In case you experience a lack of time to scan the entire research, we place this abstract at the very beginning, offering a swift overview. For projects that demand both speed and accuracy, MeshLib (MT and ST) consistently excels—combining quick processing times with robust mesh quality under heavy and moderate simplifications. If absolute geometric precision overrides speed concerns, Rhino 3D stands out for its higher fidelity (especially by Hausdorff and Average Absolute Distance metrics), albeit with slower execution. Meanwhile, CGAL could be a solid option for tasks prioritizing visual fidelity, despite its lower speed and marginally lower metrics. Finally, VTK and MeshLab Clustering Decimation should generally be avoided whenever high reliability and quality are crucial, as they frequently introduce structural issues and high error rates.
Introduction
The idea behind mesh simplification (also known as mesh decimation) is reducing the number of polygons in a given 3D mesh while preserving its overall shape and visual fidelity. Such a technique is understandably essential for optimizing 3D models. Namely, the goal here is to make meshes more suitable for real-world applications, which demand:
- Faster rendering and economical memory usage (especially in resource-constrained environments);
- Cost-efficiency which is to be secured thanks to minimized processing times and slightly reduced material consumption (say, like 3D printing);
- Compatibility based on adapting complex models for utilization on a variety of platforms.
Thus, from 3D printing and architectural visualization to medical imaging and geospatial modeling, mesh simplification underpins a wide range of use cases, making it an invaluable technique tool in computational geometry. To help you find an optimal library for mesh simplification tasks (in Python, C++, or both), our team conducted a practical search and will share some findings below.
Two Facets of Mesh Simplification
Mesh simplification is, of course, a very general term. That is to say, its practical extent varies, depending on the application. In this piece, we are going explore the following two key directions:
- Heavy mesh simplification. Here, we reduce meshes by 1000x or more (e.g., 2M to 2K triangles). This approach is typically resorted to in scenarios where speed and low resource usage are critical;
- Moderate mesh simplification, in turn, reduces meshes by 10x (e.g., 2M to 200K triangles). This balances performance with higher fidelity.
Challenges of Mesh Simplification
- Heavy mesh simplification often implies significant risks, including holes, self-intersections, and degeneracies. Such structural issues could compromise visual fidelity, disrupt rendering workflows, and lead to functional problems in manufacturing or 3D printing;
- Moderate simplification, while less extreme, presents its own pitfalls, i.e., maintaining geometric fidelity while reducing complexity. Striking the right balance between computational speed and model quality is critical to ensure the simplified surface actually remains usable.
Our Research Approach Explained
Different levels of mesh simplification are essential to match varying project needs. Understanding the trade-offs between speed and quality featured by numerous existing libraries is pivotal across both heavy and moderate cases.
We realise how difficult it is to compare libraries: you need to identify applicable ones, elaborate evaluation parameters, install, and run a testing scenario. FYI, our team took some popular libraries for comparison, from different standpoints, and contrasted them with each other for you:
Mesh Simplification Option | GitHub / Website | Primary Language(s) | Python Support | Notes |
---|---|---|---|---|
MeshLib (ST and MT) | Download on GitHub
| C++ | Yes | Provides a robust API for Python. |
CGAL | Download on GitHub
| C++ | Yes | Python bindings available via SWIG. |
LibIGL | Download on GitHub
| C++ | Yes | Offers Python bindings. |
MeshLab | Download on GitHub
| C++, JS | Yes | Python support through PyMeshLab. |
Rhino 3D | Explore the website
| C++ | Yes | Supports Python via Rhino.Python. |
Fusion 360 (Adaptive and Uniform) | Explore the website
| C++ | Yes | Unified API accessible from both Python and C++. |
3DCoat | Explore the website
| C++ | Yes | Offers both a C++ Core API and a Python API. |
Fast Quadric Mesh Simplification | Download on GitHub
| C++ | Yes | Designed for C++; Python bindings can be created through wrapping techniques. |
MeshOptimizer | Download on GitHub
| C++ | Yes | Provides Python bindings. |
VTK | Download on GitHub
| C++ | Yes | Offers Python wrappers for its functionalities. |
As for our object to run a test on, we opted for the iconic Nefertiti mesh (2M triangles) as the benchmark notable for its complexity and relevance in 3D modeling flows.
All tests were conducted on a consistent hardware basis to ensure reliable comparisons:
- Windows 11
- Intel Core i7-12700H
- 32GB RAM
- NVIDIA RTX 3060m (6GB VRAM)
Heavy Mesh Simplification Case
Again, for this test, our Nefertiti mesh (2 million triangles) was used. A reduction factor of 1000x was applied, resulting in a final mesh of 2000 triangles.
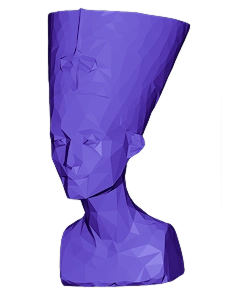
MeshLib ST
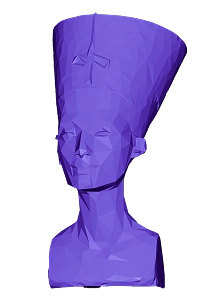
MeshLib MT
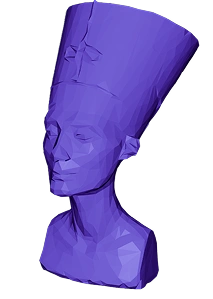
CGAL
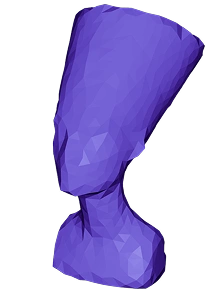
LibIGL
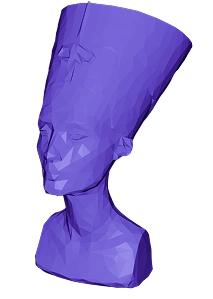
MeshLab: Quadric Edge Collapse
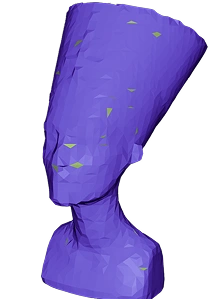
MeshLab: Clustering
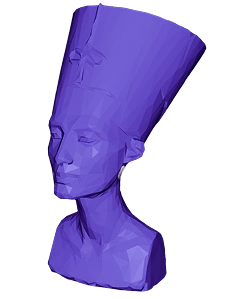
Rhino 3D
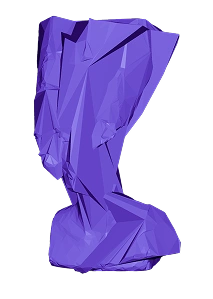
Fusion Uniform
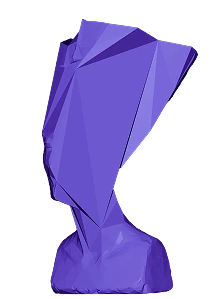
3DCoat
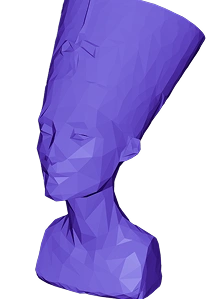
Fast Quadric Mesh Simplification
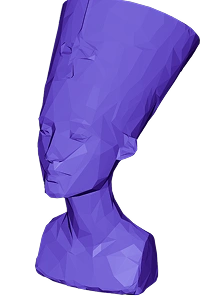
MeshOptimizer
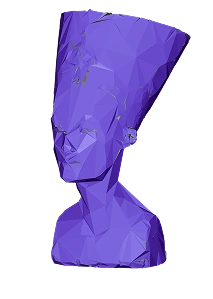
VTK
Moderate Mesh Simplification Case
For this test, our Nefertiti mesh (2 million triangles) was reduced by a factor of 10x, resulting in a final mesh of 200,000 triangles. This scenario evaluates how well libraries balance speed and quality while maintaining geometric fidelity in a less extreme reduction scenario.
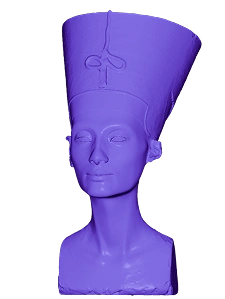
MeshLib ST
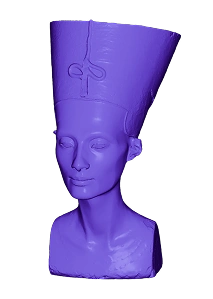
MeshLib MT
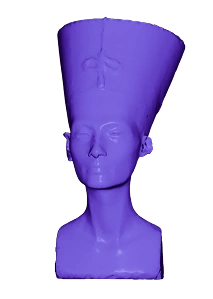
CGAL
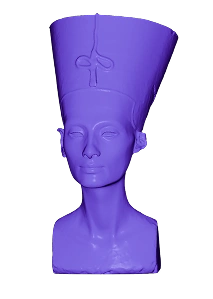
LibIGL
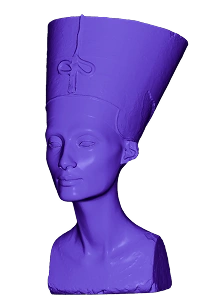
MeshLab: Quadric Edge Collapse
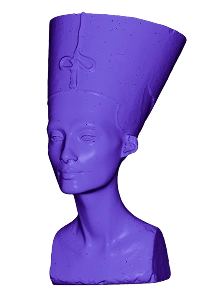
MeshLab: Clustering Decimation
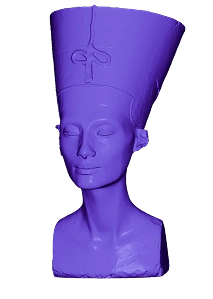
Rhino3D
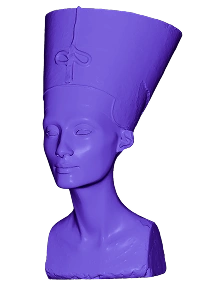
Fusion Adaptive
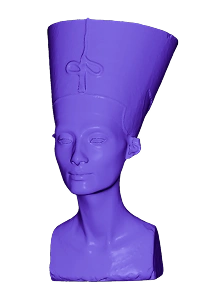
Fusion Uniform
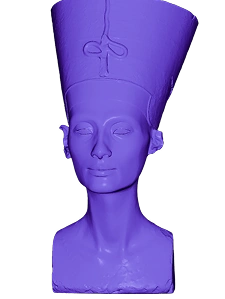
3DCoat
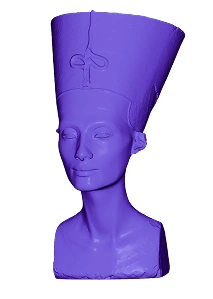
Fast Quadric Mesh Simplification
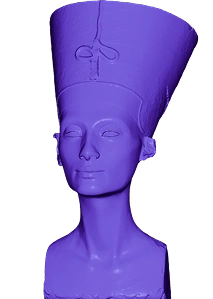
MeshOptimizer
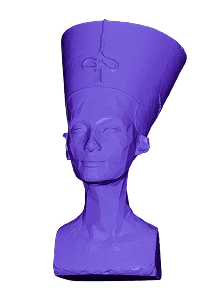
VTK
Download the Full Comparison Results Across 11 Mesh Simplification Libraries
All benchmark results, including quality comparisons and execution times across two scenarios Heavy & Moderate mesh simplification are available in a downloadable PDF.
The PDF includes:
- Execution time for each library
- Result quality side-by-side
- Observations on mesh structure, artifacts, and texture preservation
- Visual outputs
Notes on Verification Sources
In case you want to verify this research, dive deeper into it, or just feel curious, you can find all the files and code samples by following this link.
Start Your Journey with MeshLib
MeshLib SDK offers multiple ways to dive in — from live technical demos to full application trials and hands-on SDK access. No complicated setups or hidden steps. Just the tools you need to start building smarter, faster, and better.
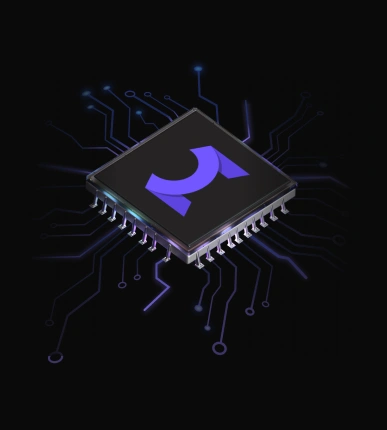